2.
LAND-COVER CLASSIFICATION AND MAPPING
Introduction
Methods
Results
Accuracy Assessment
Limitations and Discussion
Introduction
Generally, the mapping of land-cover is done by adopting or developing
a land-cover classification system, delineating areas of relative
homogeneity (basic cartographic "objects"), then labeling these
areas using categories defined by the classification system. More
detailed attributes of the individual areas are added as more information
becomes available, and a process of validating both polygon pattern
and labels is applied for editing and revising the map. This is
done in an iterative fashion, with the results from one step causing
re-evaluation of results from another step. For example, the mapping
and truthing process may reveal needed corrections to the classification
scheme. Finally, an assessment of the overall accuracy of the data
is conducted. Where the database is appropriately maintained, the
final assessment of accuracy will show where improvements should
be made in the next update (Stoms et al. 1994).
In its "coarse filter" approach to conservation biology (e.g.,
Jenkins 1985, Noss 1987), Gap Analysis relies on maps of dominant
natural land cover types as the most fundamental spatial component
of the analysis (Scott et al. 1993) for terrestrial environments.
For the purposes of GAP, most of the land surface of interest (natural)
can be characterized by its dominant vegetation.
Land cover classifications rely on specified attributes, such
as the structural features of plants, their floristic composition,
or environmental conditions, to consistently differentiate categories
(Küchler and Zonneveld 1988). The criteria for a land cover
classification system for GAP are: (a) an ability to distinguish
areas of different actual dominant vegetation; (b) a utility for
modeling vertebrate species habitats; (c) a suitability for use
within and among biogeographic regions; (d) an applicability to
Landsat Thematic Mapper (TM) imagery for both rendering a base map
and from which to extract basic patterns (GAP relies on a wide array
of information sources, TM offers a convenient mesoscale base map
in addition to being one source of actual land cover information);
(e) a framework that can interface with classification systems used
by other organizations and nations to the greatest extent possible;
and (f) a capability to fit, both categorically and spatially, with
classifications of other themes such as agricultural and built environments.
For Gap Analysis, the system that fits best is provisionally referred
to as the National Vegetation Classification Standards (NVCS). In
recent times, this system has also been referred to as the UNESCO/TNC
system (Lins and Kleckner 1996) because it is based on the structural
characteristics of vegetation derived by Mueller-Dombois and Ellenberg
(1974), adopted by the United Nations Educational, Scientific, and
Cultural Organization (UNESCO 1973) and later modified for application
to the United States by Driscoll et al. (1983, 1984). The Nature
Conservancy and the Natural Heritage Network (Grossman et al. 1994)
have been improving upon this system in recent years with partial
funding supplied by GAP. The basic assumptions and definitions for
this system have been described by Jennings (1993).
At the time the CA-GAP was developing a land-cover map, there was
no classification scheme in place that fully satisfied the NVCS.
The Nature Conservancy system of Bourgeron and Engelking (1994)
for the western United States did not include California. We chose
therefore to use the then-current classification used by the California
Natural Diversity Data Base (CNDDB) of the Natural Heritage Division
in the Department of Fish and Game (Holland 1986) for terrestrial
natural communities as a precursor to the NVCS/TNC systems. This
classification system of the CNDDB is similar to proposed NVCS alliances
in that it is based on dominant species and structure. However,
criteria for distinguishing woodland from forest, shrubland from
herbaceous, and so on, are less rigorous in the CNDDB system compared
to the very precise quantitative criteria in NVCS. Not all CNDDB
communities are named for their dominant or indicator species, such
as Sierran Mixed Coniferous Forest, but it is unlikely that NVCS
will be able to consistently label such complex communities by one
or two dominant species either. Despite these limitations and deviations
from the NVCS, the CNDDB classification has three strong advantages
for use in this generation of GAP. First, the community types are
well-described (Holland 1986) and not just listed. Second, the classification
scheme is in wide use within federal, state, regional, and local
agencies around California and therefore is well-recognized by resource
managers. Third, the Natural Heritage Division has assigned state
and global ranks to these communities, indicating their rarity and
level of threat (Keeler-Wolf 1993). Recently the CNDDB has adopted
a new classification system (Sawyer and Keeler-Wolf 1995) which
may be more in keeping with the NVCS. Future iterations of CA-GAP
may wish to cross-walk the CNDDB classes in our initial land-cover
map to this newer system.
Twelve new community types were added to the CNDDB system specifically
for CA-GAP, often based on descriptions developed by botanical experts
from various parts of the state (Appendix 2-1). Because not all
surfaces are naturally vegetated, classes for non-vegetated surfaces
such as bare ground, water, and sand dunes, plus human dominated
land uses were also defined. The standard for these classes was
based on the system developed by the USGS (Anderson et al. 1976)
as a hierarchical classification for use in remote sensing applications.
The "Anderson" system splits at the first level by land use/land
cover type into urban, agriculture, water, and barren land. The
second level was disaggregated into several agricultural types (e.g.,
cropland, orchard, vineyard), water bodies (e.g., rivers, lakes,
reservoirs), and various forms of bare ground (salt flats, beaches,
sand dunes, bare rock, and quarries or mines). Wetlands were classified
according to definitions by Cowardin et al. (1979). By this combination
of natural community types and these 27 other land use/land-cover
types, all land and water surfaces were classified in the gap analysis
database.
Methods
For CA-GAP, we identified vegetation types by one to three overstory
species, each contributing greater than 20% of relative canopy cover.
The 20% cover criterion, which we selected to be consistent with
the California Vegetation Type Mapping (VTM) survey (Wieslander
1946; see Colwell 1988, for overview), is lower than typically applied
to define canopy dominance. For example, the CALVEG classification
defines dominant as > 50% (Parker and Matyas 1981). Paysen et
al. (1980) define Series based on a single dominant overstory species
or genus. The ongoing California Native Plant Society Community
Inventory is identifying Series primarily based on a single, overstory
dominant, although a few series are based on two co-dominant species,
and others are defined by environment (e.g., Alpine Series) (Sawyer
and Keeler-Wolf 1995). For our purposes and at our 1:100,000 mapping
scale, we found that use of single canopy dominants to type vegetation
produced an unacceptable simplification of vegetation composition
and pattern. For example, much of the chaparral vegetation in the
Southwestern California Region would be mapped as Chamise or Scrub
oak chaparral, masking systematic, regional variation in community
composition. By using the 20% cover threshold, we retained information
on one to three canopy species that are dominant or co-dominant
over several-to-many hectares. This area is much larger than plot
sizes used in traditional vegetation studies. To avoid confusing
these vegetation types with Series or Associations as defined by
other systems, we refer to these combinations as Species Assemblages.
In the field, species in an assemblage may be uniformly mixed or
in a fine mosaic of patches, depending on the scale at which the
pattern is observed. This means that in practice, species assemblages
in our database can be a series recognized by existing classification
systems, a combination of two or three recognized series, or previously
unrecognized species combinations.
A map of actual vegetation was produced using summer 1990 Landsat
Thematic Mapper (TM) satellite imagery, 1990 high altitude color
infrared photography (1:58,000 scale), VTM maps based on field surveys
conducted between 1928 and 1940, and miscellaneous recent vegetation
maps and ground surveys. Details of the mapping process are provided
in Davis et al. (1995), and are only summarized here.
We did not have the resources to map individual stands of homogeneous
vegetation. Instead, we have attempted to delimit "landscapes,"
which we defined as areas of one to many square kilometers in extent
with uniform climate, physiography, substrate and disturbance regime,
and covered by a single species assemblage or by a mosaic of a few
species assemblages associated with different sites (e.g., riparian
zones, mesic slopes, xeric slopes). Landscape boundaries were mapped
subjectively by photointerpretation of patterns in the satellite
imagery. Final delineation of a landscape unit was an iterative
process based on evidence from the satellite imagery, 1990 air photos,
existing vegetation maps and field reconnaissance. The map was produced
using a minimum mapping unit of 100 ha (1 kmē). The state was mapped
into greater than 21,000 landscape units, or polygons, with a mean
size of approximately 1,950 ha.
TM imagery was resampled to the Albers equal-area projection with
100 meter resolution (i.e. 1 hectare pixels), and a false color
composite of red, near-infrared and mid-infrared reflectance images
was displayed on a video monitor. Obvious landscape boundaries were
digitally drafted over the imagery based on image tone and texture.
Ancillary information, especially air photos and VTM maps, was used
to capture additional compositional changes in vegetation that were
not visually obvious in the TM imagery. VTM maps were used to position
landscape boundaries on vegetation gradients where no obvious break
was visible on either the satellite imagery or in air photos. Two
hundred and thirty polygons (excluding urban and agricultural areas)
were checked in the field, primarily by roadside reconnaissance.
Using these various sources, a large amount of information was
collected for each landscape unit (Table 2-1). Based on our concept
of landscape, we recorded a primary species assemblage, which was
the most widespread vegetation type or land use/land cover type
in the polygon, a secondary and tertiary type, and the fraction
of the landscape covered by each type. We also recorded the most
widespread wetland assemblage, which was usually riparian vegetation.
Each species assemblage was defined by up to three dominant species.
We also recorded the occurrence of minor overstory species of special
conservation concern (e.g., Juglans californica, Quercus
engelmanii, Cupressus forbesii).
Species data were derived from field survey, air photos or from
the VTM and other detailed maps. VTM information was used for areas
where air photos provided no evidence of recent disturbance, based
on the assumption that canopy dominants observed by VTM field crews
have not changed over the past 50-60 years. We realize this is a
tenuous assumption. We found during our field surveys that the assumption
is usually valid for forest and hard chaparral types. Although the
relative dominance of species may have changed over the interval,
species that were mapped as co-dominants by VTM crews in the 1930s
are usually still canopy dominants across the same landscape. The
composition of shrubland and grassland types is not as stable over
the same interval, and we made special efforts to view these types
in the field or to find more current maps. Our landscape units are
many square kilometers in extent, and canopy composition can vary
greatly from site to site within a landscape. Thus the species assemblages
that we have mapped record those species that most frequently dominate
most sites in that landscape.
Table 2-1. Landscape attributes for CA-GAP land-cover
map units.
Polygon ID Number
Primary Vegetation
Dominant Species 1
Codominant Species 2
Codominant Species 3
Canopy Closure (4 classes)
Fraction of polygon occupied by primary type (10% intervals)
CNDDB Community type
WHR habitat type
Secondary Vegetation
Dominant Species 1
Co-dominant Species 2
Codominant Species 3
Canopy Closure
Fraction of polygon occupied by type
CNDDB Community type
WHR habitat type
Tertiary Vegetation
Dominant Species 1
Co-dominant Species 2
Codominant Species 3
Canopy Closure
Fraction of polygon occupied by type
CNDDB Community type
WHR habitat type
Presence/Absence of 9 wetland habitat types (CA WHR types)
Primary Wetland Vegetation
Dominant Species 1
Codominant Species 2
Codominant Species 3
Presence of canopy species of special status (narrow endemics,
RTE species)
We have tried to account for fire dynamics by recording recent
burns and by retaining information on the pre-burn dominants (e.g.,
an area of recently burned chamise chaparral that is presently dominated
by herbs would be recorded as sparse chamise canopy co-dominated
by annual herbs).
Rather than a multi-colored vegetation map, the information we
have developed is better treated as a vegetation database linked
to a set of areas. One can retrieve distribution data on individual
species, unique combinations of species, or vegetation types defined
by physiognomy and/or composition (Stoms et al. 1992, Davis et al.
1995). Although the database approach provides a more flexible framework
for representing vegetational variation than the traditional vegetation
map, it does not eliminate the need for classification in order
to simplify and communicate results. We recorded many thousands
of unique species (or species/landuse) combinations in over 21,000
polygons. Many unusual species combinations occurred at the margins
of regions in transitional environments. We summarize distribution
data for individual dominant species and based on plant communities
as defined in the California NDDB (Holland 1986), which we derived
from the database by an equivalence table assigning each species
combination to a unique NDDB community. The criteria for class assignment
in the NDDB classification system are qualitative and often not
explicitly based on dominant overstory species. Where ambiguities
existed, we assigned species combinations to more general types.
For example, Holland (1986) identified several pinyon and/or juniper
community types in the Mojave Desert region that we aggregated into
a single type at his next higher level of classification.
Several procedures were implemented to ensure quality in the final
map. The distribution of each dominant plant species in the coverage
was compared to the documented distribution recorded in the CalFlora
database (see http://s27w007.pswfs.gov/calflora/) which was derived
from the Munz flora and revised with some more current data. Apparent
outlying locations of species in the GAP database were re-examined
to either confirm that the location was documented in the data source
or to change the species code if it appeared to be an incorrect
interpretation or a data input error. A similar comparison was made
for each community type with the written description in Holland
(1986).
Results
Of the entire state, 323,492 kmē (79%) was classified as vegetation
other than agricultural or urban land uses, water, or bare ground.
13% is mapped as various forms of agriculture, with an additional
4.5% in urban or industrial uses. Mean size of the 21,171 landscape
units is 1,930 ha, with a median size of 528 ha. The relative proportions
of map units by size class is shown in Figure 2-1.
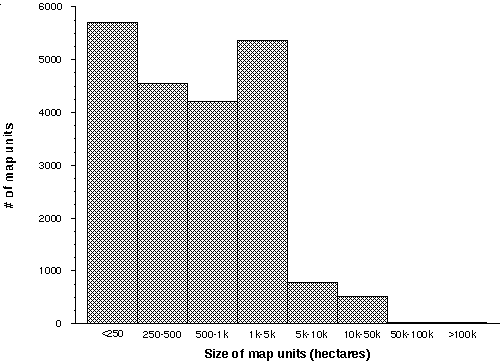
Figure 2-1. Frequency of the size of map units in
the CA-GAP land-cover map.
The statewide land-cover map contains distributional information
on 221 different land use/cover classes. Of these, 182 were original
CNDDB community types described by Holland (1986) or on the California
Department of Fish & Game's August, 1987, updated list. In addition,
12 new community types and 27 land use/land cover types were added
specifically for CA-GAP. The database contains distributional information
on 96 trees, 237 shrubs, 56 herbaceous species or groups of species
where they appear as dominants in the canopy (i.e., at least 20%
cover in a community type).
Despite the large number of natural communities mapped for the
CA-GAP, eight types are particularly widespread (>10,000 kmē
each), collectively representing nearly 45% of the vegetated land
in California (Table 3-2). These types, in descending order of extent,
include Mojave Creosote Bush Scrub (34100), Non-native Grassland
(42200), Sierran Mixed Coniferous Forest (84230), Coast Range Mixed
Coniferous Forest (84110), Sonoran Creosote Bush Scrub (33100),
Mojave Mixed Woody Scrub (34210), Blue Oak Woodland (71140), and
Foothill Pine-Oak Woodland (71410). On the other hand, 37 types
were mapped over less than 25 kmē each. Some of these types are
indeed rare, and their distributions may be well-known and depicted
accurately in the GAP land-cover database (e.g., Monvero Residual
Dunes, Valley Sacaton Grassland). Other types, particularly the
wetland and riparian communities, may be more widespread than indicated
in Table 2-2, but generally occur in small patches below the resolution
of the GAP database.
Table 2-2. Total area and percentage of each land-cover
type in California.
Land-Cover Code |
Land-Cover Type |
Total Mapped Distribution (kmē) |
% of State |
|
Native Plant Communities |
|
|
21210 |
Northern
Foredunes |
3.4 |
<0.1 |
21310 |
Northern
Dune Scrub |
106.9 |
<0.1 |
21320 |
Central
Dune Scrub |
13.6 |
<0.1 |
22000 |
Desert
Dunes |
2,155.1 |
0.5 |
23300 |
Monvero
Residual Dunes |
3.0 |
<0.1 |
31100 |
Northern
Coastal Bluff Scrub |
39.1 |
<0.1 |
31200 |
Southern
Coastal Bluff Scrub |
22.6 |
<0.1 |
32100 |
Northern
(Franciscan) Coastal Scrub |
469.0 |
0.1 |
32200 |
Central
(Lucian) Coastal Scrub |
680.5 |
0.2 |
32300 |
Venturan
Coastal Sage Scrub |
2,102.1 |
0.5 |
32500 |
Diegan
Coastal Sage Scrub |
1,316.9 |
0.3 |
32600 |
Diablan
Sage Scrub |
1,107.3 |
0.3 |
32700 |
Riversidian
Sage Scrub |
744.3 |
0.2 |
33100 |
Sonoran
Creosote Bush Scrub |
12,625.1 |
3.1 |
33200 |
Sonoran
Desert Mixed Scrub |
6,323.9 |
1.5 |
34100 |
Mojave
Creosote Bush Scrub |
43,711.6 |
10.7 |
34210 |
Mojave
Mixed Woody Scrub |
10,542.3 |
2.6 |
34220 |
Mojave
Mixed Steppe |
643.7 |
0.2 |
34240 |
Mojave
Mixed Woody and Succulent Scrub |
574.6 |
0.1 |
34300 |
Blackbush
Scrub |
1,796.6 |
0.4 |
35100 |
Great
Basin Mixed Scrub |
6,391.2 |
1.6 |
35110 |
Salvia
dorri/ Chamaebatiaria scrub * |
30.4 |
<0.1 |
35210 |
Big
Sagebrush Scrub |
4,341.2 |
1.1 |
35211 |
Low
Sagebrush Scrub * |
1,623.8 |
0.4 |
35212 |
Silver
Sagebrush Scrub * |
431.0 |
0.1 |
35213 |
Black
Sagebrush Scrub * |
18.2 |
<0.1 |
35220 |
Subalpine
Sagebrush Scrub |
171.4 |
<0.1 |
35400 |
Rabbitbrush
Scrub |
419.1 |
0.1 |
35500 |
Cercocarpus
ledifolius Woodland * |
828.2 |
0.2 |
36110 |
Desert
Saltbush Scrub |
4,723.2 |
1.2 |
36120 |
Desert
Sink Scrub |
697.4 |
0.2 |
36130 |
Desert
Greasewood Scrub |
829.6 |
0.2 |
36140 |
Shadscale
Scrub |
3,492.3 |
0.9 |
36150 |
Desert
Holly Scrub * |
1,448.0 |
0.4 |
36210 |
Valley
Sink Scrub |
250.4 |
0.1 |
36220 |
Valley
Saltbush Scrub |
1,915.5 |
0.5 |
36320 |
Interior
Coast Range Saltbush Scrub |
27.7 |
<0.1 |
37110 |
Northern
Mixed Chaparral |
1,652.4 |
0.4 |
37120 |
Southern
Mixed Chaparral |
219.4 |
0.1 |
37200 |
Chamise
Chaparral |
5,381.4 |
1.3 |
37300 |
Red
Shank Chaparral |
965.6 |
0.2 |
37400 |
Semi-Desert
Chaparral |
2,462.4 |
0.6 |
37510 |
Mixed
Montane Chaparral |
1,852.0 |
0.5 |
37520 |
Montane
Manzanita Chaparral |
1,329.0 |
0.3 |
37530 |
Montane
Ceanothus Chaparral |
1,083.6 |
0.3 |
37541 |
Shin
Oak Brush |
202.9 |
<0.1 |
37542 |
Huckleberry
Oak Chaparral |
287.4 |
0.1 |
37550 |
Bush
Chinquapin Chaparral |
190.4 |
<0.1 |
37610 |
Mixed
Serpentine Chaparral |
217.2 |
0.1 |
37620 |
Leather
Oak Chaparral |
98.4 |
<0.1 |
37810 |
Buck
Brush Chaparral |
4,196.3 |
1.0 |
37820 |
Blue
Brush Chaparral |
74.3 |
<0.1 |
37830 |
Ceanothus
crassifolius Chaparral |
2,092.6 |
0.5 |
37840 |
Ceanothus
megacarpus Chaparral |
583.0 |
0.1 |
37900 |
Scrub
Oak Chaparral |
2,162.0 |
0.5 |
37A00 |
Interior
Live Oak Chaparral |
2,105.1 |
0.5 |
37B00 |
Upper
Sonoran Manzanita Chaparral |
836.3 |
0.2 |
37C10 |
Northern
Maritime Chaparral |
2.9 |
<0.1 |
37C20 |
Central
Maritime Chaparral |
218.0 |
0.1 |
37D00 |
Ione
Chaparral |
0.8 |
<0.1 |
37E00 |
Mesic
North Slope Chaparral |
628.3 |
0.2 |
37G00 |
Coastal
Sage-Chaparral Scrub |
345.4 |
0.1 |
39000 |
Upper
Sonoran Subshrub Scrub |
375.2 |
0.1 |
41000 |
Coastal
Prairie |
880.3 |
0.2 |
42110 |
Valley
Needlegrass Grassland |
7.4 |
<0.1 |
42120 |
Valley
Sacaton Grassland |
9.1 |
<0.1 |
42160 |
Desert
Native Grassland * |
300.3 |
0.1 |
42200 |
Non-Native
Grassland |
27,483.4 |
6.7 |
42300 |
Wildflower
Field |
7.0 |
<0.1 |
43000 |
Great
Basin Grassland |
117.9 |
<0.1 |
44110 |
Northern
Hardpan Vernal Pool |
0.1 |
<0.1 |
44120 |
Northern
Claypan Vernal Pool |
1.6 |
<0.1 |
44131 |
Northern
Basalt Flow Vernal Pool |
3.9 |
<0.1 |
45100 |
Montane
Meadow |
360.5 |
0.1 |
45200 |
Subalpine
or Alpine Meadow |
285.5 |
0.1 |
45310 |
Alkali
Meadow |
477.0 |
0.1 |
45500 |
Great
Basin Wet Meadow * |
197.0 |
<0.1 |
46000 |
Alkali
Playa |
1,436.5 |
0.4 |
47000 |
Pavement
Plain |
11.3 |
<0.1 |
51110 |
Sphagnum
Bog |
1.1 |
<0.1 |
52110 |
Northern
Coastal Salt Marsh |
84.7 |
<0.1 |
52120 |
Southern
Coastal Salt Marsh |
11.0 |
<0.1 |
52200 |
Coastal
Brackish Marsh |
310.8 |
0.1 |
52310 |
Cismontane
Alkali Marsh |
24.5 |
<0.1 |
52320 |
Transmontane
Alkali Marsh |
33.8 |
<0.1 |
52410 |
Coastal
and Valley Freshwater Marsh |
501.3 |
0.1 |
52420 |
Transmontane
Freshwater Marsh |
305.8 |
0.1 |
61110 |
North
Coast Black Cottonwood Riparian Forest |
11.9 |
<0.1 |
61130 |
Red
Alder Riparian Forest |
20.3 |
<0.1 |
61210 |
Central
Coast Cottonwood-Sycamore Riparian Forest |
77.6 |
<0.1 |
61220 |
Central
Coast Live Oak Riparian Forest |
29.2 |
<0.1 |
61230 |
Central
Coast Arroyo Willow Riparian Forest |
42.5 |
<0.1 |
61310 |
Southern
Coast Live Oak Riparian Forest |
26.0 |
<0.1 |
61320 |
Southern
Arroyo Willow Riparian Forest |
37.7 |
<0.1 |
61330 |
Southern
Cottonwood-Willow Riparian Forest |
59.9 |
<0.1 |
61410 |
Great
Valley Cottonwood Riparian Forest |
330.5 |
0.1 |
61420 |
Great
Valley Mixed Riparian Forest |
73.3 |
<0.1 |
61430 |
Great
Valley Valley Oak Riparian Forest |
91.6 |
<0.1 |
61510 |
White
Alder Riparian Forest |
27.5 |
<0.1 |
61520 |
Aspen
Riparian Forest |
59.4 |
<0.1 |
61530 |
Montane
Black Cottonwood Riparian Forest |
25.8 |
<0.1 |
61610 |
Modoc-Great
Basin Cottonwood-Willow Riparian Forest |
80.3 |
<0.1 |
61700 |
Mojave
Riparian Forest |
58.8 |
<0.1 |
61810 |
Sonoran
Cottonwood-Willow Riparian Forest |
2.7 |
<0.1 |
61820 |
Mesquite
Bosque |
273.9 |
0.1 |
62100 |
Sycamore
Alluvial Woodland |
11.3 |
<0.1 |
62200 |
Desert
Dry Wash Woodland |
3,003.7 |
0.7 |
62400 |
Southern
Sycamore-Alder Riparian Woodland |
18.1 |
<0.1 |
63100 |
North
Coast Riparian Scrub |
99.3 |
<0.1 |
63200 |
Central
Coast Riparian Scrub |
2.1 |
<0.1 |
63310 |
Mule
Fat Scrub |
74.7 |
<0.1 |
63320 |
Southern
Willow Scrub |
1.6 |
<0.1 |
63330 |
Southern
Alluvial Fan Scrub * |
13.2 |
<0.1 |
63410 |
Great
Valley Willow Scrub |
18.9 |
<0.1 |
63420 |
Great
Valley Mesquite Scrub |
22.6 |
<0.1 |
63500 |
Montane
Riparian Scrub |
119.9 |
<0.1 |
63600 |
Modoc-Great
Basin Riparian Scrub |
84.6 |
<0.1 |
63700 |
Mojave
Desert Wash Scrub |
2.0 |
<0.1 |
63810 |
Tamarisk
Scrub |
105.1 |
<0.1 |
63820 |
Arrowweed
Scrub |
13.4 |
<0.1 |
71110 |
Oregon
Oak Woodland |
2,436.9 |
0.6 |
71120 |
Black
Oak Woodland |
1,641.4 |
0.4 |
71130 |
Valley
Oak Woodland |
922.0 |
0.2 |
71140 |
Blue
Oak Woodland |
10,451.8 |
2.6 |
71150 |
Interior
Live Oak Woodland |
1,731.6 |
0.4 |
71160 |
Coast
Live Oak Woodland |
1,172.2 |
0.3 |
71170 |
Alvord
Oak Woodland |
339.7 |
0.1 |
71182 |
Dense
Engelmann Oak Woodland |
234.8 |
0.1 |
71210 |
California
Walnut Woodland |
58.5 |
<0.1 |
71310 |
Open
Foothill Pine Woodland |
1,495.8 |
0.4 |
71321 |
Serpentine
Foothill Pine-Chaparral Woodland |
836.7 |
0.2 |
71322 |
Non-Serpentine
Foothill Pine Woodland |
787.8 |
0.2 |
71410 |
Foothill
Pine-Oak Woodland |
10,180.5 |
2.5 |
71420 |
Mixed
North Slope Cismontane Woodland |
1,005.3 |
0.2 |
71430 |
Juniper-Oak
Cismontane Woodland |
301.8 |
0.1 |
71600 |
Oak-Piñon
Woodland * |
135.8 |
<0.1 |
72100 |
Great
Basin Woodlands |
9,157.8 |
2.2 |
72200 |
Mojavean
Pinyon and Juniper Woodlands |
4,035.6 |
1.0 |
72300 |
Peninsular
Pinyon and Juniper Woodlands |
480.0 |
0.1 |
72400 |
Cismontane
Juniper Woodland and Scrub |
31.2 |
<0.1 |
73000 |
Joshua
Tree Woodland |
123.3 |
<0.1 |
81100 |
Mixed
Evergreen Forest |
4,646.5 |
1.1 |
81200 |
California
Bay Forest |
13.2 |
<0.1 |
81310 |
Coast
Live Oak Forest |
2,193.7 |
0.5 |
81320 |
Canyon
Live Oak Forest |
1,736.2 |
0.4 |
81330 |
Interior
Live Oak Forest |
2,708.9 |
0.7 |
81340 |
Black
Oak Forest |
5,614.9 |
1.4 |
81400 |
Tan-Oak
Forest |
1,824.7 |
0.4 |
81B00 |
Aspen
Forest |
187.8 |
<0.1 |
82100 |
Sitka
Spruce-Grand Fir Forest |
339.3 |
0.1 |
82310 |
Alluvial
Redwood Forest |
742.0 |
0.2 |
82320 |
Upland
Redwood Forest |
5,407.6 |
1.3 |
82410 |
Coastal
Douglas-Fir-Western Hemlock Forest |
32.4 |
<0.1 |
82420 |
Upland
Douglas-Fir Forest |
344.3 |
0.1 |
82500 |
Port
Orford Cedar Forest |
5.5 |
<0.1 |
83110 |
Beach
Pine Forest |
14.0 |
<0.1 |
83120 |
Bishop
Pine Forest |
180.6 |
<0.1 |
83130 |
Monterey
Pine Forest |
39.2 |
<0.1 |
83161 |
Mendocino
Pygmy Cypress Forest |
10.5 |
<0.1 |
83210 |
Knobcone
Pine Forest |
341.6 |
3.8 |
83220 |
Northern
Interior Cypress Forest |
182.9 |
<0.1 |
83330 |
Southern
Interior Cypress Forest |
20.5 |
<0.1 |
84110 |
Coast
Range Mixed Coniferous Forest |
13,539.2 |
3.3 |
84120 |
Santa
Lucia Fir Forest |
8.4 |
<0.1 |
84130 |
Coast
Range Ponderosa Pine Forest |
468.9 |
0.1 |
84140 |
Coulter
Pine Forest |
405.2 |
0.1 |
84150 |
Bigcone
Spruce-Canyon Oak Forest |
341.6 |
0.1 |
84160 |
Ultramafic
White Pine Forest |
22.2 |
<0.1 |
84171 |
Northern
Ultramafic Jeffrey Pine Forest |
496.3 |
0.1 |
84180 |
Ultramafic
Mixed Coniferous Forest |
262.2 |
0.1 |
84210 |
Westside
Ponderosa Pine Forest |
9,750.3 |
2.4 |
84220 |
Eastside
Ponderosa Pine Forest |
6,382.7 |
1.6 |
84230 |
Sierran
Mixed Coniferous Forest |
15,637.1 |
3.8 |
84240 |
Sierran
White Fir Forest |
1,055.8 |
0.3 |
84250 |
Big
Tree Forest |
171.3 |
<0.1 |
84260 |
Modoc
White Fir Forest * |
942.7 |
0.2 |
85100 |
Jeffrey
Pine Forest |
3,257.7 |
0.8 |
85120 |
Red
Fir-Western White Pine Forest * |
1,598.8 |
0.4 |
85210 |
Jeffrey
Pine-Fir Forest |
4,308.7 |
1.1 |
85310 |
Red
Fir Forest |
4,475.7 |
1.1 |
85320 |
Southern
California White Fir Forest |
38.1 |
<0.1 |
85410 |
Siskiyou
Enriched Coniferous Forest |
245.1 |
0.1 |
85420 |
Salmon-Scott
Enriched Coniferous Forest |
1,047.1 |
0.3 |
86100 |
Lodgepole
Pine Forest |
2,572.3 |
0.6 |
86210 |
Whitebark
Pine-Mountain Hemlock Forest |
429.4 |
0.1 |
86220 |
Whitebark
Pine-Lodgepole Pine Forest |
588.2 |
0.1 |
86300 |
Foxtail
Pine Forest |
238.0 |
0.1 |
86400 |
Bristlecone
Pine Forest |
99.1 |
<0.1 |
86500 |
Southern
California Subalpine Forest |
52.4 |
<0.1 |
86600 |
Whitebark
Pine Forest |
233.3 |
0.1 |
86700 |
Limber
Pine Forest |
21.0 |
<0.1 |
91110 |
Klamath-Cascade
Fell-field |
59.0 |
<0.1 |
91120 |
Sierra
Nevada Fell-field |
122.5 |
<0.1 |
94000 |
Alpine
Dwarf Scrub |
611.0 |
0.1 |
|
Types Other Than Native Plant Communities |
|
|
11100 |
Urban
or Built-Up Land |
18,352.5 |
4.5 |
11200-11213 |
Agricultural
types |
52,426.3 |
12.9 |
11300 |
Eucalyptus
Groves (exotic) |
34.8 |
<0.1 |
11401-11402 |
Conifer
Plantations |
1,335.8 |
0.3 |
11510 |
Streams
and Canals |
352.8 |
0.1 |
11520 |
Permanently-flooded
Lacustrine Habitat |
4,059.3 |
1.0 |
11521 |
Intermittently-flooded
Lacustrine Habitat |
223.1 |
0.1 |
11540 |
Bays
and Estuaries |
221.1 |
0.1 |
11710 |
Dry
Salt Flat |
866.1 |
0.2 |
11720 |
Beaches
and Coastal Dunes |
73.1 |
<0.1 |
11730 |
Sandy
Area Other than Beaches |
171.3 |
<0.1 |
11740 |
Bare
Exposed Rock |
5,875.2 |
1.4 |
11750 |
Strip
Mines, Quarries and Gravel Pits |
247.4 |
0.1 |
11760 |
Transitional
Bare Areas |
101.3 |
<0.1 |
11770 |
Mixed
Barren Land |
651.1 |
0.2 |
11780 |
Mud
Flats |
154.5 |
<0.1 |
|
Statewide
Total - All Lands |
408,636 |
100.0 |
The statewide land-cover map also contains information on the distribution
of 59 wildlife habitat types as described by the California Wildlife-Habitat
Relationships System (Mayer and Laudenslayer 1988). Of these, 45
types are native upland or wetland habitats, 11 are various agricultural
land uses, one represents all urban areas, and 2 are open water
habitats in rivers and lakes. The method for assigning habitat types
to map units based on the combinations of dominant plant species
is described in detail in Chapter 3 as part of the wildlife distribution
modeling. Because the focus of GAP is on inventorying natural ecosystems,
agricultural habitats in the database are only indicative of the
dominant agricultural use of land. Where data were available that
distinguised cultivated types at the level of WHR habitats (e.g.,
deciduous vs. evergreen orchards), this information was used. In
many cases, however, the specific types could not be readily determined
and were labeled as a more general category such as Orchard and
Vineyard (OVN) or even the most general Cropland (CRP). Also the
CWHR classification of agricultural habitats was refined midway
through the CA-GAP mapping effort. Data recorded prior to this classification
revision were not revised. Therefore, agricultural habitats in southern
California are particularly likely to be coded as more general types.
Of the 45 native habitats, 8 account for 46% of the total land
area in the state (Table 2-3). The most widespread type is Desert
Scrub (over 17%). Annual Grassland is second most common (7.1%).
Other common habitats are Alkali Desert Scrub, Ponderosa Pine, Sierran
Mixed Conifer, Mixed Chaparral, Blue Oak-Foothill Pine Woodland,
and Sagebrush, all with between 4.1 and 3.3 percent of the area
of California. The least common types, as mapped by CA-GAP, are
Saline Emergent Wetland and Palm Oasis, with less than 0.1% each.
Table 2-3. Total area and percentage of each wildlife
habitat type in California.
WHR Code |
Wildlife Habitat Type (Mayer and Laudenslayer
1988) |
Total Mapped Distribution (kmē) |
% of State |
|
Native Habitat Types |
|
|
SCN |
Subalpine
Conifer |
3,891 |
1.0 |
RFR |
Red
Fir |
5,787 |
1.4 |
LPN |
Lodgepole
Pine |
2,097 |
0.5 |
SMC |
Sierran
Mixed Conifer |
14,606 |
3.6 |
WFR |
White
Fir |
2,935 |
0.7 |
KMC |
Klamath
Mixed Conifer |
5,137 |
1.3 |
DFR |
Douglas-Fir |
4,239 |
1.0 |
JPN |
Jeffrey
Pine |
7,980 |
2.0 |
PPN |
Ponderosa
Pine |
16,385 |
4.0 |
EPN |
Eastside
Pine |
4,690 |
1.1 |
RDW |
Redwood |
6,517 |
1.6 |
PJN |
Pinyon-Juniper |
6,359 |
1.6 |
JUN |
Juniper |
8,210 |
2.0 |
ASP |
Aspen |
249 |
0.1 |
CPC |
Closed-cone
Pine-Cypress |
1,233 |
0.3 |
MHC |
Montane
Hardwood-Conifer |
10,349 |
2.5 |
MHW |
Montane
Hardwood |
11,118 |
2.7 |
BOW |
Blue
Oak Woodland |
10,579 |
2.6 |
VOW |
Valley
Oak Woodland |
3,130 |
0.8 |
COW |
Coastal
Oak Woodland |
4,419 |
1.1 |
BOP |
Blue
Oak-Foothill Pine |
14,400 |
3.5 |
MRI |
Montane
Riparian |
524 |
0.1 |
VRI |
Valley-Foothill
Riparian |
800 |
0.2 |
DRI |
Desert
Riparian |
363 |
0.1 |
POS |
Palm
Oasis |
7 |
<0.1 |
JST |
Joshua
Tree |
379 |
0.1 |
ADS |
Alpine-Dwarf
Shrub |
755 |
0.2 |
LSG |
Low
Sage |
2,778 |
0.7 |
BBR |
Bitterbrush |
218 |
0.1 |
SGB |
Sagebrush |
13,668 |
3.3 |
MCP |
Montane
Chaparral |
3,104 |
0.8 |
MCH |
Mixed
Chaparral |
14,300 |
3.5 |
CRC |
Chamise-Redshank
Chaparral |
10,498 |
2.6 |
CSC |
Coastal
Scrub |
7,598 |
1.9 |
DSS |
Desert
Succulent Shrub |
4,459 |
1.1 |
DSW |
Desert
Wash |
3,017 |
0.7 |
DSC |
Desert
Scrub |
70,343 |
17.2 |
ASC |
Alkali
Desert Scrub |
16,885 |
4.1 |
AGS |
Annual
Grassland |
28,921 |
7.1 |
PGS |
Perennial
Grassland |
477 |
0.1 |
WTM |
Wet
Meadow |
672 |
0.2 |
FEW |
Freshwater
Emergent Wetland |
1,317 |
0.3 |
SEW |
Saline
Emergent Wetland |
136 |
<0.1 |
EST |
Estuarine |
216 |
0.1 |
BAR |
Barren |
8,269 |
2.0 |
|
Developed, Cultivated or Water Habitats |
|
|
URB |
Urban |
18,378 |
4.5 |
|
Agricultural
types |
51,620 |
12.6 |
RIV |
Riverine |
354 |
0.1 |
|
Statewide
Total - All Lands |
408,636 |
100.0 |
Accuracy Assessment
GAP land-cover maps are primarily compiled to answer the fundamental
question in gap analysis: what is the current distribution and management
status of the nation's major natural land cover types and wildlife
habitats? Besides giving a measure of overall reliability of the
land cover map for Gap Analysis, the assessment will also need to
identify which general classes or which regions of the map do not
meet the accuracy objectives for the Gap Analysis Program. Thus
the assessment identifies where additional effort will be required
when the map is updated. We report the results of the accuracy assessment,
believing that the map is the best statewide map currently available.
Gap Analysis land-cover maps have already been used in local and
regional conservation planning and will likely be used in coordinated
national interagency land cover assessments. The maps should be
useful for biogeographic analyses and as input to mesoscale models
of climate and ecosystem processes. They may well be used to assess
the accuracy of smaller-scale land cover maps. These secondary users
also need to know the per class accuracy of the maps. Some users
would also benefit from having some measure of accuracy by polygon
or geographic area, such as where the map is most reliable. We expect
that in general, secondary users will need more details about the
accuracy assessment to make appropriate use of the map than will
primary users who are more familiar with the data.
The purpose of accuracy assessment is to allow a potential user
to determine the map's "fitness for use" for their application.
It is impossible for the original cartographer to anticipate all
future applications of a land cover map, so the assessment should
provide enough information for the user to evaluate fitness for
their unique purpose. This can be described as the degree to which
the data quality characteristics collectively suit an intended application.
The information reported include details on the database's spatial,
thematic, and temporal characteristics and their accuracy.
Assessment data are valuable for purposes beyond their immediate
application to estimating accuracy of a land cover map. The reference
data is therefore made available to other agencies and organizations
for use in their own land cover characterization and map accuracy
assessments (see Data Use and Availability for access information).
The data set will also serve as an important training data source
for later updates.
Even though we have reached an endpoint in the mapping process
where products are made available to others, the Gap Analysis process
should be considered dynamic. We envision that maps will be refined
and updated on a regular schedule. The assessment data will be used
to refine Gap Analysis maps iteratively by identifying where the
land cover map is inaccurate and where more effort is required to
bring the maps up to accuracy standards. In addition, the field
sampling may identify new classes that were not identified at all
during the initial mapping process.
Map accuracy can be assessed in many different ways, most commonly
by comparing the map to ground observations for a set of sample
"points" (Congalton 1991). This approach is not practical for small
scale maps such as ours because of the sampling effort required
to determine the actual map class at a point on the ground when
the minimum mapping unit is 1 kmē. The size and limited accessibility
of some parts of the study area also pose considerable financial
and logistical challenges. For these reasons, we have not conducted
a formal assessment of the accuracy of the vegetation database.
Instead, we have attempted to provide a qualitative measure of map
accuracy through roadside reconnaissance and by comparing our map
with recent, detailed vegetation maps that have been prepared for
parts of the region. Because our approach varied among regions,
additional information on map accuracy is deferred to individual
regional summaries in the appendix. In general, our studies indicate
that the vegetation database has inaccuracies but is generally in
high agreement with other, recent vegetation maps (Stine et al.
1996, Stoms 1996, Franklin and Woodcock 1997). However, it is a
highly generalized abstraction of vegetation pattern that can serve
only for broad regional assessments and inventories. The database
will be maintained by the California Department of Fish and Game,
and we fully anticipate that the map will undergo periodic revision
based on feedback from botanical experts.
Limitations and Discussion
Gap analysis
provides a regional overview of the distribution and ownership profile
of major terrestrial plant communities and vertebrate species habitats.
It is not a substitute for a detailed biological inventory. Our
assessment focuses on floristically (and to some extent, structurally)
defined plant community types and wildlife habitat types and does
not account for variations in stand age or physical stature within
a type. For example, we do not distinguish late seral/old growth
forest from younger forest of the same general community type.
The extent and
spatial scale of the land-cover map make a formal, statistical analysis
of map accuracy impractical for both financial and logistical reasons.
As a result, we cannot with confidence place error terms on our
estimates of area or management status of plant communities.
The method that
we used to map land-cover is not suited to the analysis of most
wetland types or other communities that are restricted to very localized
environments. The mapping method is well suited to analysis of shrubs
and trees, but it provides little or no information on the distribution
of herbaceous species. Our analyses assume that the vegetation or
habitat types attributed to a map unit (polygon) are dispersed uniformly
throughout the unit.
Estimates of
area made from maps are very sensitive to map scale and mapping
methods. For example, vegetation types that typically occur in small
patches may be overlooked or their extent underestimated using a
vegetation map with relatively coarse spatial resolution. Our vegetation
map is less sensitive to spatial resolution than traditional paper
maps because we maintain database records of secondary and tertiary
vegetation types that are too fine to map using a 100 ha (247 ac)
mmu. The point to remember is that our estimates of the acreage
and distributions of species and types may differ considerably from
areal estimates and distributions of the same types derived from
maps prepared at a finer or coarser resolution.
The static nature
of the gap analysis data also limits their utility in assessing
conservation risk. Our database provides a snapshot of large regions
within which land-cover is very dynamic. Thus the database does
not reflect how much of a community type may have been lost due
to past human land uses nor what proportion is likely to be converted
or degraded in the future.
|